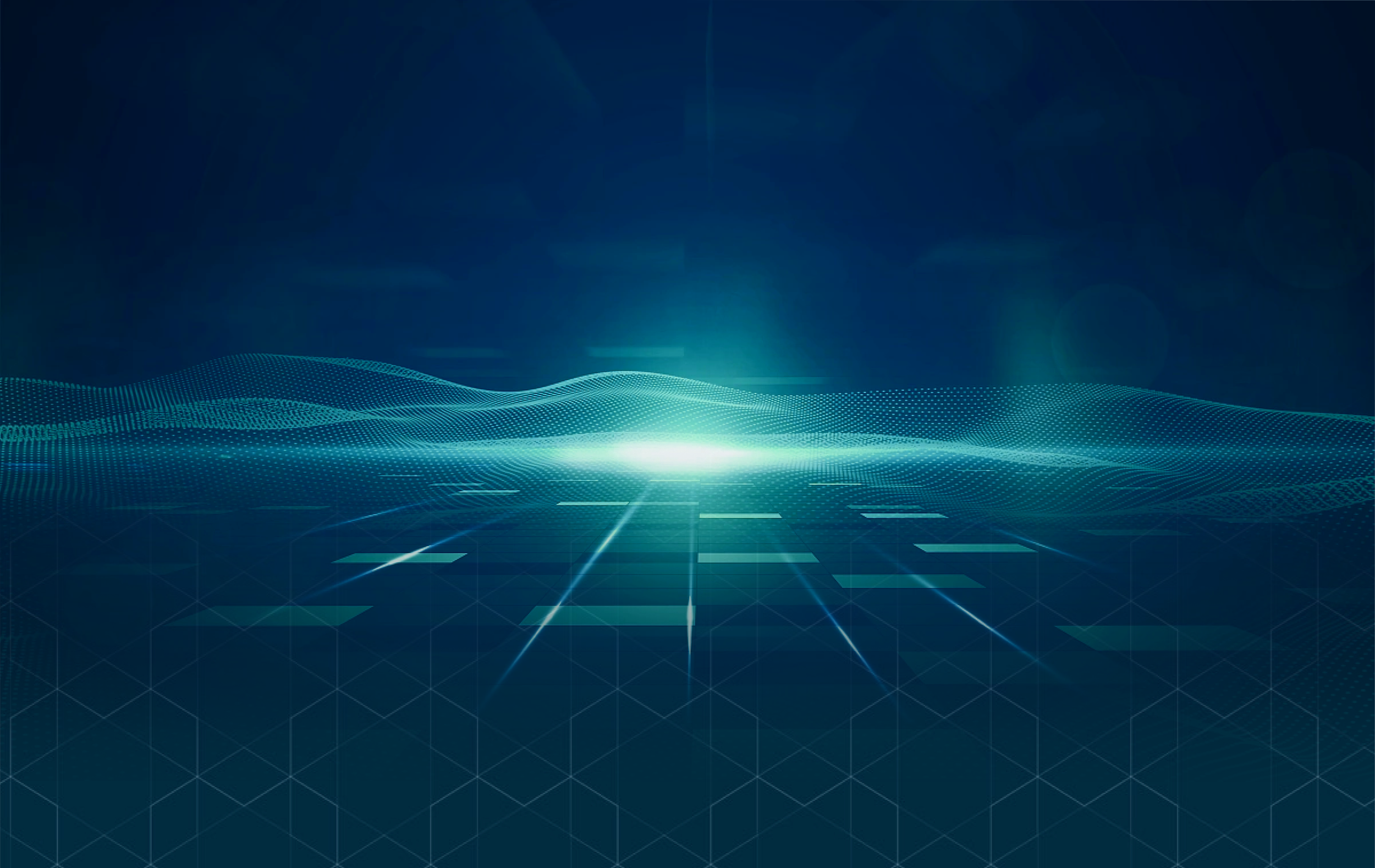
Causal AI Features
-
Causal Discovery
-
Causal Inference
-
Integration with Domain Knowledge
-
Decision-making
Automated Root Cause Inference: Unveiling Cause and Effect with Causal Reasoning
Autonomously detecting anomalies, Ari automates the root cause analysis in aid to decision-making. As shown in the video on the right, upon identifying the 'High condenser approach temperature' fault in an industrial cooling water system, Ari runs causal reasoning in an autonomous manner to pinpoint the root cause in a causal graph discovered with causal machine learning. In seamless integration with LLMs, conclusions of the root cause reasoning can be generated in a conversational agent which responds to queries with relevant and accurate answers.
Automatically Establish Cause and Effect with Machine Learning
R&B's causal engine integrated with LLMs is capable of performing autonomous causal discovery across various structured and unstructured datasets. With a reasonable amount of effort in preprocessing structured data, the engine will automatically uncover causal relationships between variables using a hybrid approach of unsupervised and supervised machine learning methods. A causal graph will be generated to represent the distinct variables (or datasets) and the inferred causal connections with quantitative measurement on causality. An alternative to establish causal relationships especially in a domain is to leverage the ability of LLMs to discover causality in a qualitative manner.
Causal inference.
When the causal discovery is completed the engine will start executing causal inference in which causal factors are investigated to decide how they influence, in another word, cause specific outcomes with quantitative measurements.